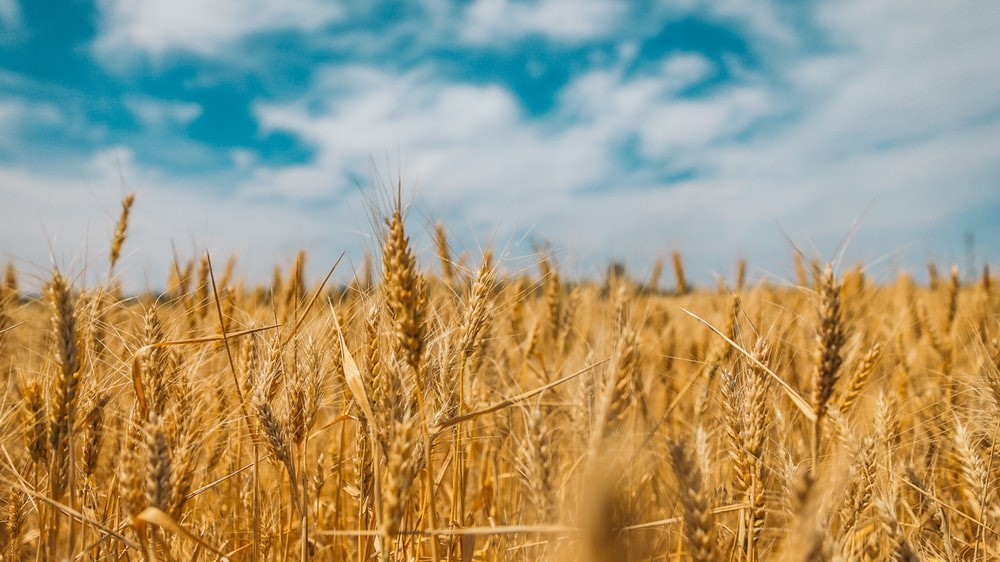
22 Jul EARLY PREDICTION OF WHEAT YIELD BASED ON RGB AND THERMAL IMAGES USING DEEP LEARNING
Željana Grbović
Junior Researcher, Institute BioSense
Wheat is one of the most important crops in food production worldwide. Due to the population increase, it is necessary to boost production and supplies both in wheat and other cereals.
Year 2019 marks the third consecutive season that wheat was observed in different stages on the experimental fields of BioSense Institute. The images were taken by FLIR SC620 camera (Figure 1) in the first two seasons in oblique perspective and by mobile phone mounted with a thermal camera (Figure 2) in the last season in bird eye perspective.
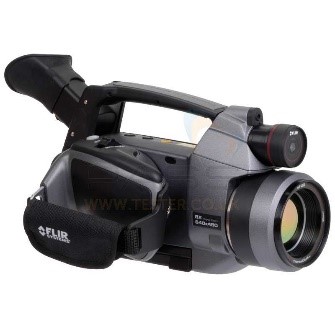
Figure 1. FLIR SC620 camera
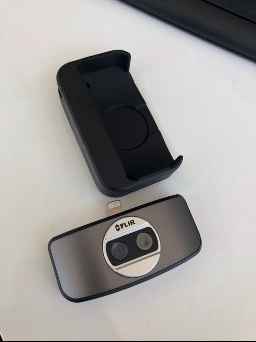
Figure 2. FLIR ONE PRO for iOS
The goal of these experiments is to enable the farmer to take a picture of the wheat growing in their field (four weeks before harvest), upload it through a mobile application, and gain information about the wheat yield estimate based on the current state of growth. If the estimated yield is below average, it will be possible to timely apply agronomic treatments to improve yield. The automatization of ear density calculation (number of ears per unit ground area, usually 1m2), which is one of the main agronomic yield components in determining grain yield in wheat, can provide fast evaluation of this attribute and potentially save 200 hours of manual work, ease monitoring, and increase crop management practice efficiency. This will save money from potential yield reduction, which can cause big losses in the farmers’ investments.
The currently used process of yield prediction includes manual and tedious work. The farmer takes samples from the area of 1m2 from the field (if the field is larger, then from a few locations within a field), and measures the biomass. The next step is to separate and count the ears of wheat manually. Since the counting of one sample requires up to 1 hour, while the number of samples can easily exceed 200, this can result in more than 200 working hours, or two to three weeks of manual labor that could be avoided.
The collected dataset comprises RGB and thermal images. Thermal images give us information about the difference in temperature between the ears and their background through their coloring and ease ear detection. Images were taken in four dates on two locations in two stages of wheat growth.
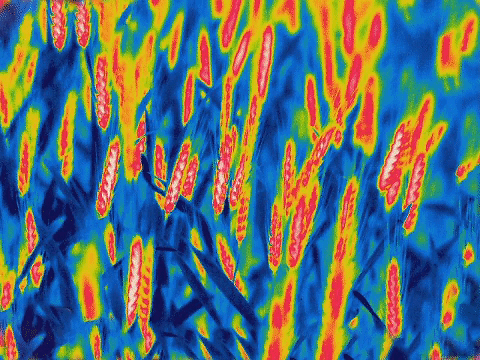
Figure 3. Thermal image
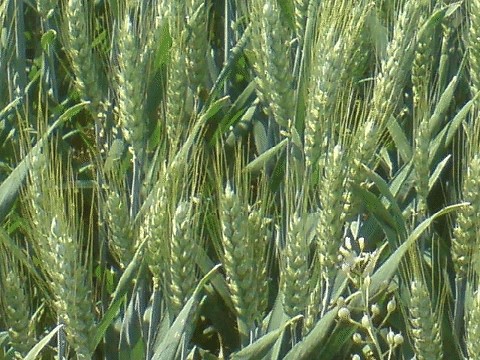
Figure 4. RGB image
Since we have witnessed a huge breakthrough of neural networks, especially in image processing, deep learning has greatly outperformed classical models and algorithms. The nature of deep learning is that the addition of more data improves the quality of results, so by uploading images from farmers (crowdsourcing), the initial database will be expanded, so the algorithm will achieve better and more accurate results.
Within the research, a trade-off between demanding memory usage, time of model training and model performances has been discussed. Three activation functions were compared: Rectified Linear Unit (ReLU), Parametrized Rectified Linear Unit (PReLU) and Exponential Linear Units (ELU).
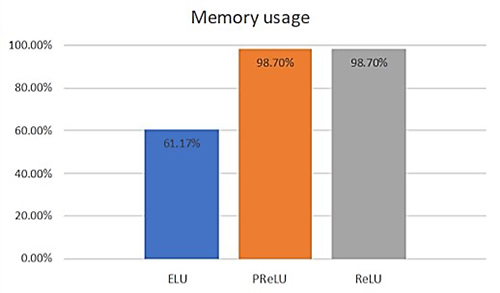
Figure 5. Memory usage
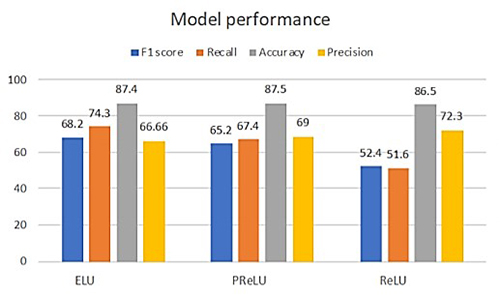
Figure 6. Model performance
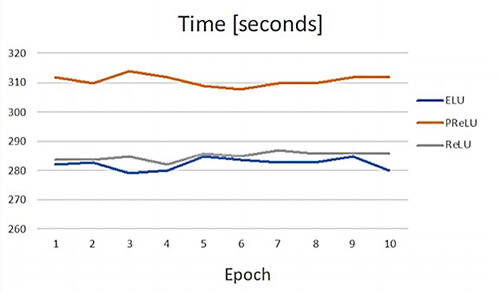
Figure 7. Time analysis
ELU outperformed other two activation functions on required memory usage and model training time with no impact or reducing model performance (Figure 1, 2 and 3).
The algorithm reached an accuracy of 92% and an F1 score of 75% of corrected segmented pixels in thermal images, and 67% and 68% in RGB. The average segmentation error, represented as the number of ears, is five in a thermal, and none in RGB images. The algorithm for automatic counting ears has miscounted in the average three ears for both sets of images. The algorithm is accurate 89% for thermal and 82% for RGB. Summarized performance of the algorithm for automatic ears counting is presented in Figure 8.
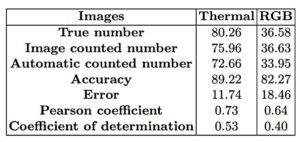
Figure 8. The algorithm for automatic counting ears performance
Future work is directed towards reducing the occlusion and overlapping, which is the most often cause of segmentation error. Also, the differences in the ear sizes require some alignment. Besides, it is inevitable to do image acquisition in more controlled conditions.
Even though this approach is workable for smaller fields, the idea is to ease the labor-intensive practice for data inquiry. The methodology could be developed for modern mechanization through an application and sensors. Novel applications developed by researchers at BioSense Institute are dedicated to make precise agriculture a wide-accepted practice, providing even small farm holders to benefit from IT in agriculture.