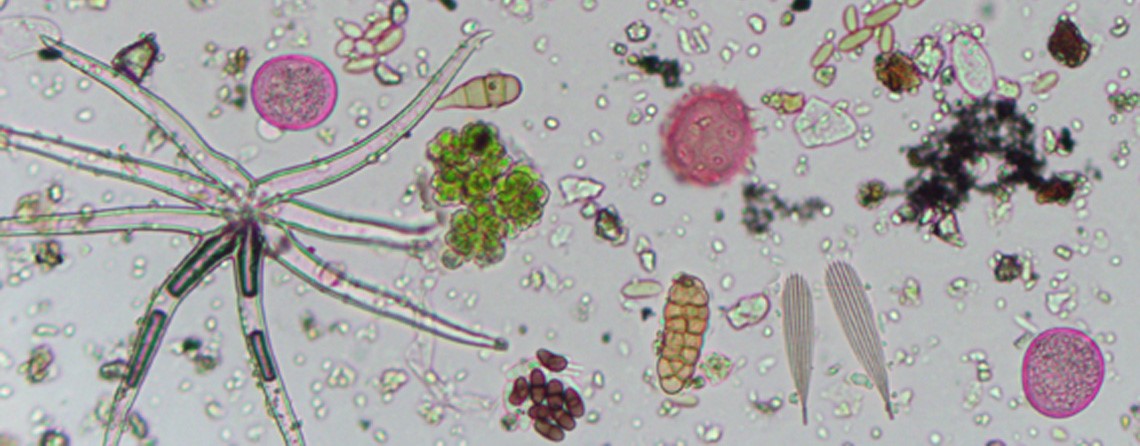
12 Sep Real-time pollen detection and identification for successful prevention of seasonal allergies symptoms
Predrag Matavulj
Junior Researcher, Institute BioSense
Pollen is one of the most common triggers of seasonal allergies. Most of the pollen that causes allergic reactions comes from trees, weeds, and grasses. When they begin to blossom, quality of life significantly decreases for people sensitive to pollen. More than 100 million Europeans suffer from allergic rhinitis and 70 million from asthma (European Parliament’s 2013 Declaration), which is estimated to cost the European Union 50-150 billion euros per year. The appropriate information on the number of airborne allergens is a prerequisite for the prevention of allergy symptoms, but also an assessment of the effectiveness of the therapy, which significantly reduces the negative health and economic impact of this widespread non-communicable disease.
The diversity of aerosols we inhale, magnified 400 times under a light microscope is presented in Figure 1. Besides pollen grains (colored pink and red), there are spores of mold, plant hair, algae, scales from butterfly wings and soot particles. Many of these particles have a bad health impact because they can cause disease in humans, animals or plants.
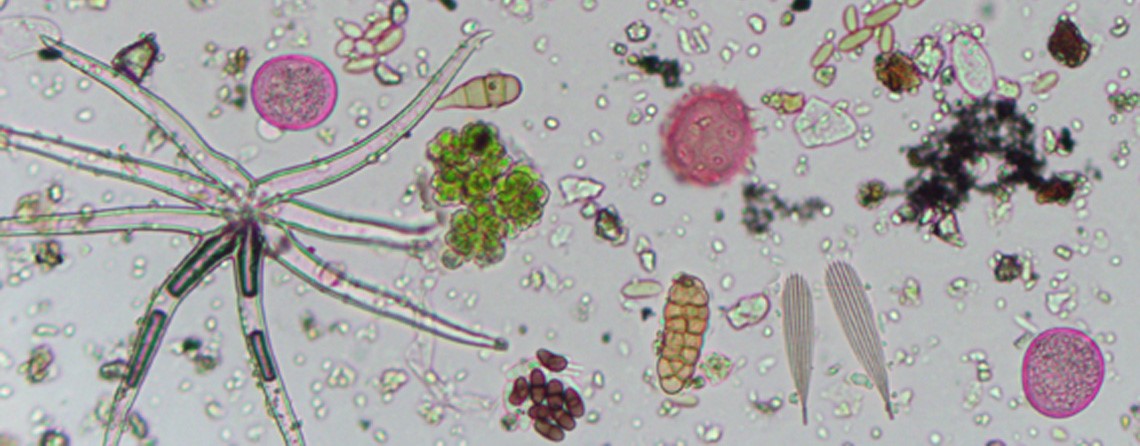
Figure 1. The diversity of aerosols present in the air.
The detection and pollen quantification are still done manually with a time-consuming microscopic analysis, based on morphology examination, so the information about the atmospheric pollen concentrations reaches the users with a delay that’s sometimes longer than seven days. Therefore, there is exigency for fast, reliable and cost-effective aerosol detectors, which will enable the availability of information on atmospheric concentrations of allergens in real-time. New laser-based technology – Rapid-E – promises to deliver real-time information using a flow cytometry technique by recording scattered light and laser-induced fluorescence patterns, representing morphological and chemical fingerprints of airborne particles.
As the particle passes through the detection chamber, it is illuminated by a laser beam. The dispersed photons are collected by detectors at different angles to the direction of the arrival of the laser beam. In this way, a picture of the light scattering of each particle is formed.
In Figure 2 you can see the signal of the laser beam scattering from a pollen grain at which the variation in the intensity of the pixels, both at the angles of detection (y-axis) and during the recording time (x-axis), reflects the shape and size of the detected particle. Since the size and shape can be extremely variable in nature, the chemical analysis allows additional discrimination between the detected particles. The chemical characteristics of the aerosol are deduced from the fluorescence spectra after the excitation using the laser with a wavelength of 337nm. For pollen of biological origin, the fluorescence spectrum results from the presence of the most significant biomolecules (e.g. flavins, nicotinamide dinucleotide, aromatic amino acids). In order to increase the possibility of discrimination between different bioaerosols, the device is also recording the rate of decrease in the intensity of the fluorescence after the dual excitation of the laser.
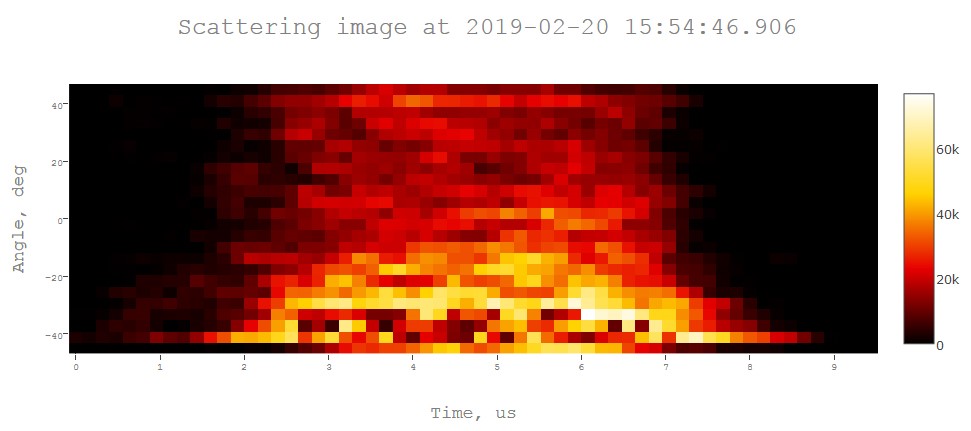
Figure 2. Signal of the laser beam scattering from a pollen grain.
Using artificial intelligence, this complex multidimensional signal is analyzed and compared with a reference signal in order to identify the type of aerosol in real-time. Artificial neural networks are one of the main tools for data science. As the “neuron” part of their name suggests, these systems are inspired by the brain and the goal of neural networks is to replicate the way people learn. The fluorescence spectrum, lifetime, and scattered light images are utilized in Convolutional Neural Network (CNN) for airborne pollen identification. The CNN first extracts the features from the data, fuses them, and then the classification is performed. This allows the neuronal weight updates for each individual source. Figure 3 illustrates this process, from the detection of particles to data analysis using artificial neural networks.
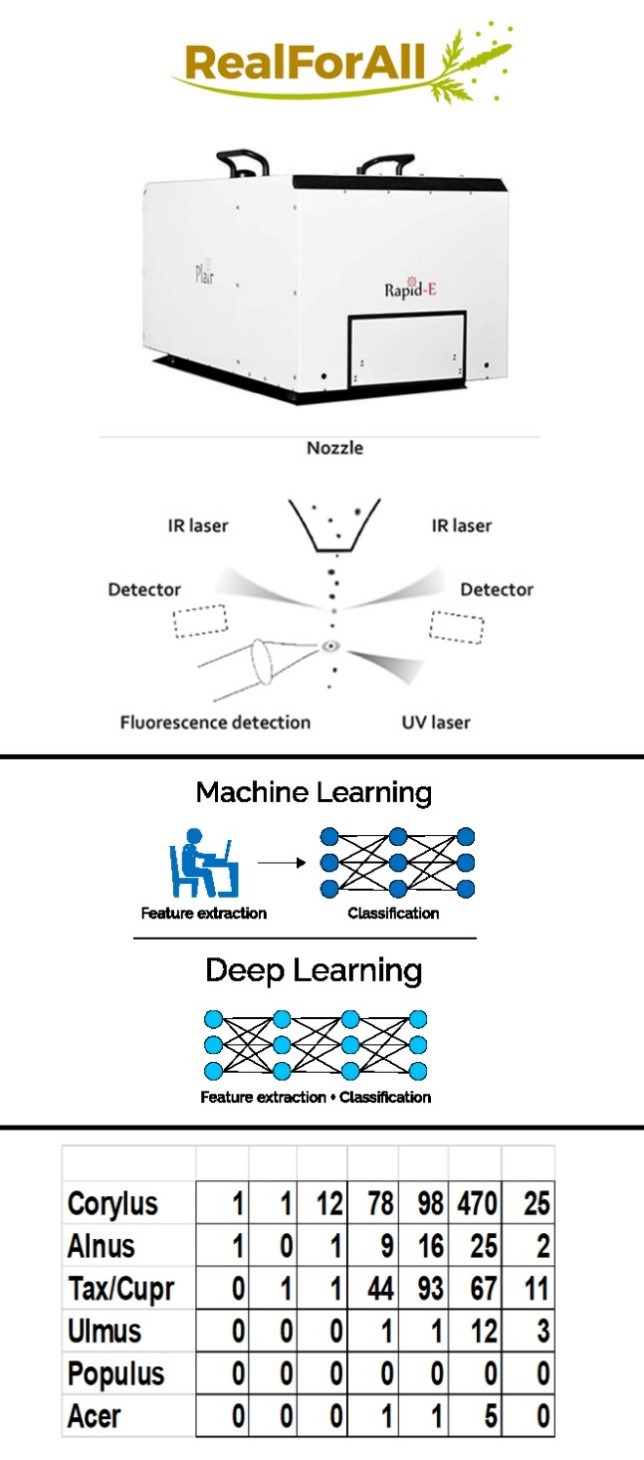
Figure 3. From the sampling of aerosols to the concentrations of allergenic airborne pollen by using flow cytometry and artificial neural networks.
Digitalization in aerobiology aims to improve the prevention and treatment of diseases. The information is delivered to the end-users via RealForAll application, whereby each smartphone becomes a unique tool for monitoring the intensity of symptoms in relation to exposure to allergens. In this way, communication between the patient and the doctor is improved, and confidence in the efficiency of treatment is increased, which is a prerequisite for a high level of compliance with the prescribed therapy. In addition to the impact on public health (seasonal allergy), there is a possibility of using atmospheric diagnostics in the protection of plants (fungal diseases), which will lead to the optimal use of medicines/pesticides and thus reduce the costs and harmful effects of treatments. Moreover, the pollen produced by plants can be an indicator of crop productivity and therefore can be used as a yield prediction method.